Churchill Barriers, Orkney
Did you know that Orkney is made up of over 70 different islands? Many of these are uninhabited. Alongside Orkney’s rich Neolithic history, more recent historical events have shaped the Orkney islands we know today.
Completed in 1945 to protect the eastern approaches to Scapa Flow naval anchorage, the Churchill Barriers are causeways which today connect four of Orkney’s islands by road: Lamb Holm, Glimps Holm, Burray and South Ronaldsay.
Due to their exposed location, road access to the Churchill Barriers can be restricted under certain conditions:
- Sea mist
- Sea spray
- Wave overtopping
What are the challenges of modelling flood hazards for the Churchill Barriers?
The Churchill Barriers are exposed to complex metocean conditions impacted by Arctic, North Sea and North Atlantic weather systems. These systems create complicated wave and wind conditions which, combined with submersed shipwrecks, means that modelling wave overtopping in this context is extremely challenging. Adding in the layer of subjective, on-the-ground decision-making during storm events, provides a component that cannot be accounted for through physics.
In 2018 SEPA developed a new flood forecasting system for Orkney. Typically these systems rely on offline deterministic modelling to predict wave overtopping under a range of event scenarios. When live, the system then matches these to the short-term forecast conditions including waves, sea level and wind, to predict whether overtopping is likely.
Improving flood and coastal hazard forecasting through Machine Learning
JBA Labs supported a pilot project to explore the possibility of supplementing traditional flood and overtopping forecasting at the Barriers by harnessing machine learning. The model is built completely based on past decision-making at the Barriers to understand when road closures were implemented.
Information about Barrier closures can be found in locally issued alerts:
X: OIC Roads (@OIC_Roads) / X
This was combined with the available wave and wind re-analysis data from the Copernicus Marine Service and ERA5 hindcast dataset from the European Centre for Medium-Range Weather Forecasts' (ECMWF) - to train the machine learning model to understand under what conditions a decision was made (or not) to close the road. This added information allows us to more accurately predict when the road should close due to extreme events compared to more traditional deterministic thresholding methods.
How do we train the model?
Training a machine learning model involves carefully adjusting a multitude of parameters, much like fine-tuning the dials on a complex control panel, to achieve the most accurate predictions possible. This pilot project took a three-stage approach:
Training the model: Using real-life data including when the barriers were open/closed, and what wind/wave conditions caused the closure.
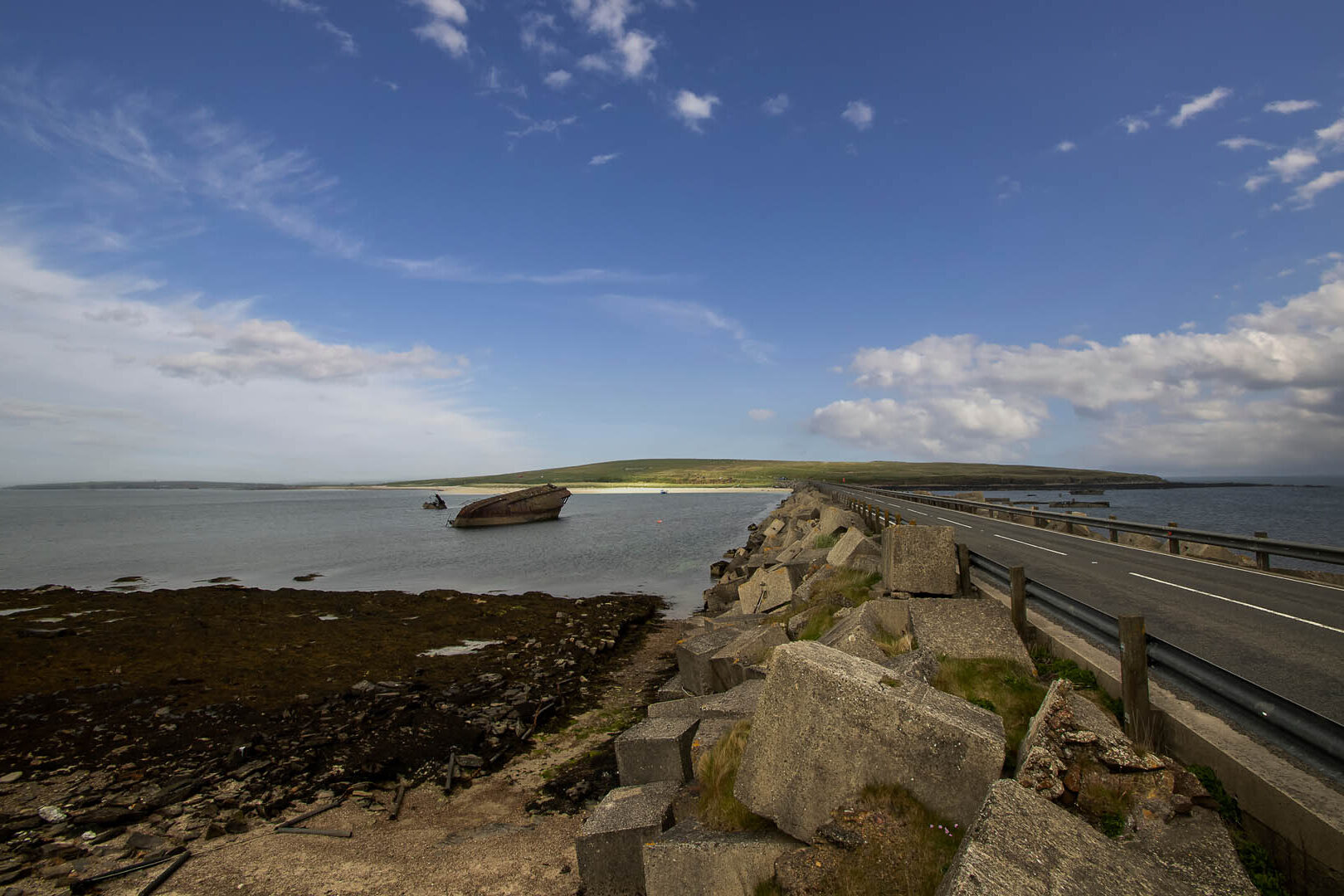
Validating the model: Blind testing the model to make a prediction based on the wave and wind conditions label data, and then checking to see if the model forecast correctly.
Testing the model: Using data that the model has never seen before to assess that it is forecasting as accurately as possible. This phase is important as it is what would be expected from a live, real-world operational forecasting system.
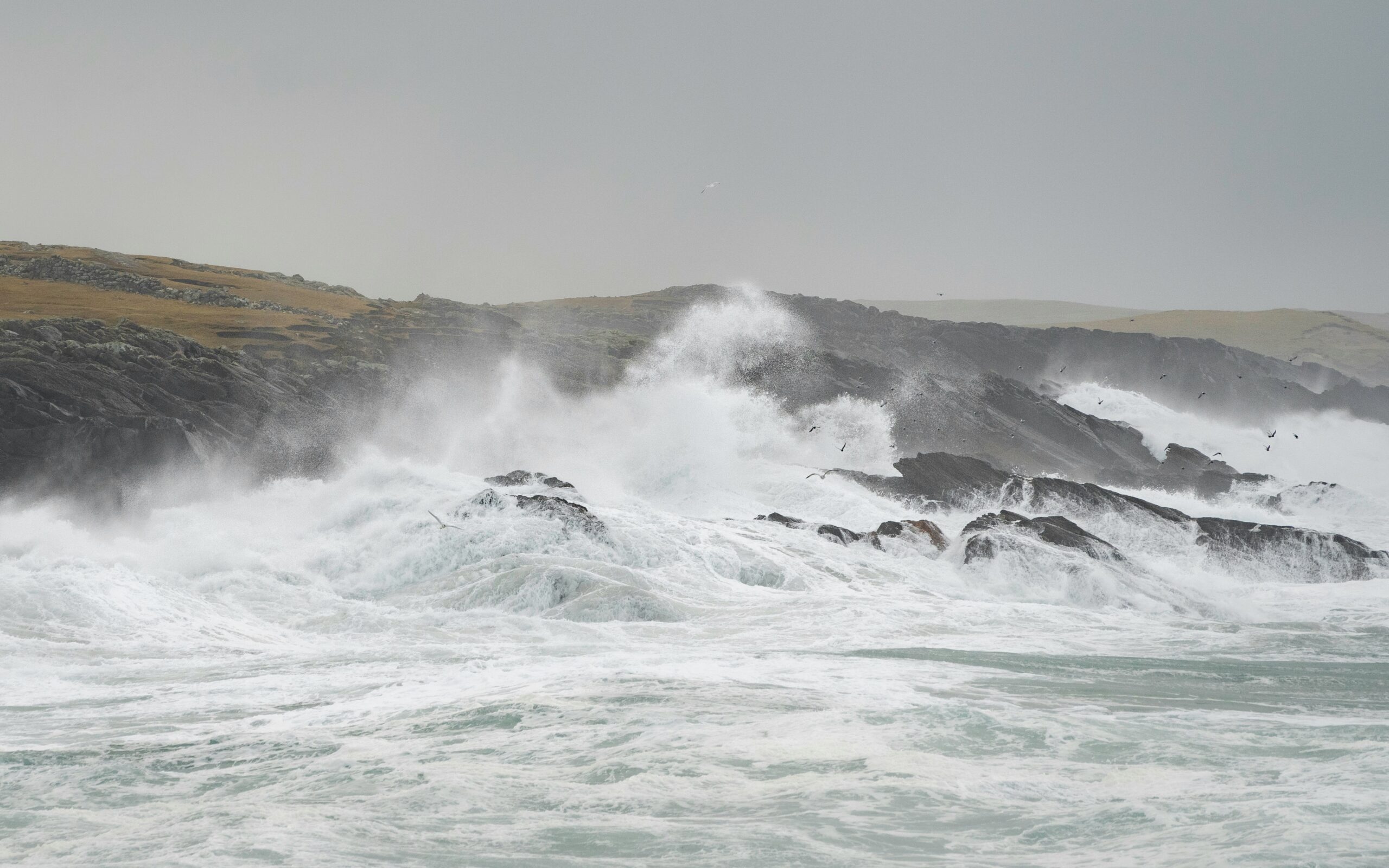
What next?
As a JBA Labs pilot scheme, we’re excited to see the potential of this machine learning model and to understand how the model performs in the context of forecasting metocean conditions for the Churchill Barriers. Our current flood model can be used to forecast the potential need for road closures due to wave overtopping.
However, the model doesn’t extend to forecasting sea mist or sea-spray inundation conditions.
Machine learning offers a way to advance our current forecasting capacity as well as bringing opportunities to subjective decision-making problems such as transportation and emergency services.
Contact Daniel Tudor for more information about this project: Harnessing machine learning to optimise coastal hazard forecasting